Tips For Publishing Your Machine Learning Study
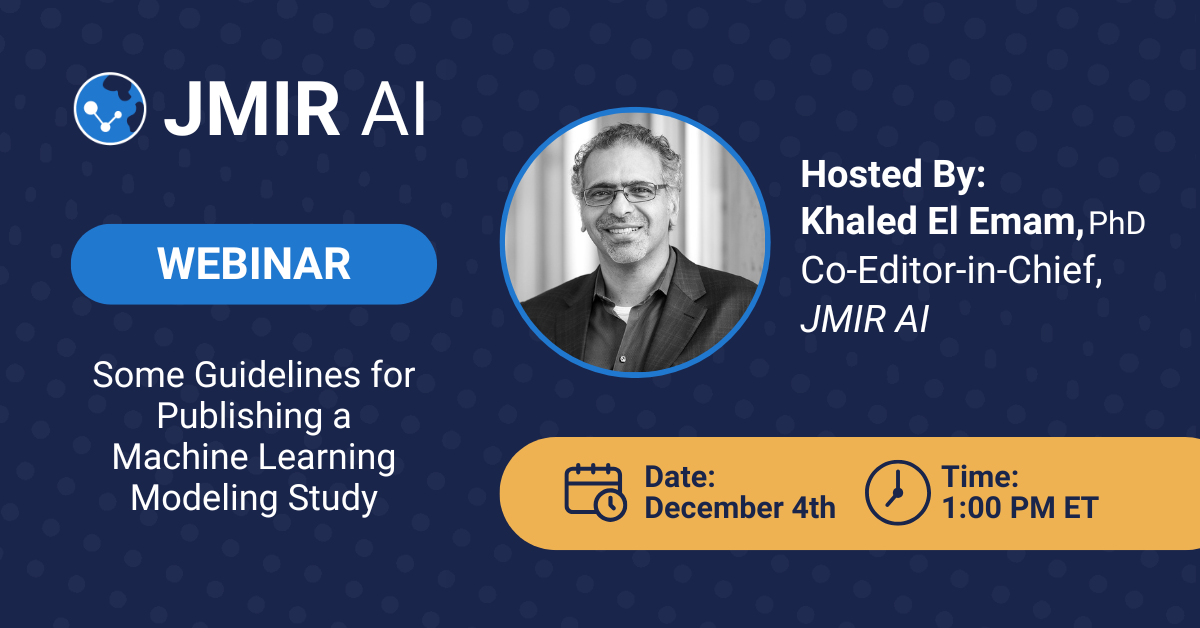
The world of machine learning research is rapidly evolving, and with it, the need for clear and concise reporting standards. Recently, the JMIR AI journal hosted a webinar titled "Guidelines for Publishing Machine Learning Modeling Studies," featuring Dr. Khaled El Emam, Co-Editor-in-Chief of JMIR AI. This blog post summarizes the key takeaways for researchers looking to publish their machine learning modeling studies.
Watch The Webinar Recording
Why Guidelines Matter
Following established guidelines ensures your research is clear, reproducible, and ultimately more impactful. Reviewers can easily assess the quality and validity of your work, leading to smoother submissions and faster publication times.
Read Some of Dr.Khaled El Emam’s Research
Key Points Covered in the Webinar
- Following Reporting Guidelines: Dr. El Emam stressed the importance of adhering to established guidelines, such as those provided by JMIR AI. These guidelines ensure all essential information is included, enhancing the reproducibility of your research.
- Justifying Data Sources and Ethics Reviews: Be transparent about the origin of your data sets and explain any ethics reviews associated with their collection. If no ethics review was required, clearly state the reason.
- Defining Clear Inclusion/Exclusion Criteria: Clearly define the criteria used to include or exclude observations in your study. This helps readers understand your sample population and the generalizability of your findings.
- Establishing Baselines for Evaluation: Compare the performance of your model to a relevant baseline. This helps put your results in context and determine the actual value of your model.
- Justifying Predictor Selection: Explain the rationale behind your choice of features and the cutoffs used for predicted probabilities. Remember, features should be readily available for collection and use in real-world applications.
- Avoiding Data Leakage: Data leakage occurs when information from the testing set influences the training process, leading to artificially inflated performance. Careful data handling practices are crucial to avoid this pitfall. Common scenarios discussed included handling multiple observations per patient, imbalanced data sets, and missing data imputation.
Actionable Tips
- Document Hyperparameters: Carefully document the hyperparameters used in your model training and justify your choices, including any tuning procedures.
- Consider External Validation: While not yet a requirement, external validation of your model on a separate dataset can further strengthen your findings.
- Embrace Synthetic Data: Dr. Al Imam suggested using synthetic data augmentation for both research and privacy protection purposes.
- Unsupervised Learning: For unsupervised learning projects, refer to the EQUATOR Network guidelines for reporting best practices.
Looking Forward
Clear and transparent reporting is essential for advancing the field of machine learning research. By following established guidelines and addressing the points highlighted in this webinar, researchers can significantly improve the quality and impact of their published work.
We encourage you to explore these resources and reach out to JMIR AI with any questions you may have. Stay tuned for upcoming events and publications by following JMIR AI and JMIR Publications on social media.
Subscribe Now